Can the Generative AI Beast Ever Be Tamed?
Spoiler alert: No!
Aside from all the hype (and investment) that generative AI is attracting, it is important to take a step back and ask a few fundamental questions:
- How much ROI can investors expect – realistically?
- What can and can’t generative AI do?
- How feasible is deploying generative AI in production, and at scale?
Right now, the cost of training large AI models outweigh the return by a massive margin (NVIDIA being the exception). OpenAI has already burned the $10B investment from Microsoft and is flirting with the autocratic Gulf sheikdoms to bridge the gap.
So, why are investors racing to throw billions at pretty much any startup with “AI” on its banner (most of which simply build wrappers for ChatGPT APIs)?
In my opinion, it’s precisely because the expectations can’t be measured! From an investor’s POV, this means that the potentials are infinite. Perhaps “FOMO” fits the bill here.
This also answers the second question about which productivity problems generative AI can solve, reliably of course. Apple have recently made bold announcements about their upcoming killer AI, but ended up approaching Google to integrate Gemini into their locked ecosystem! Unprecedented.
The Transformer architecture has surely made it easy for anyone with a deep pocket to train large generative models. However, perfecting the models seems far fetched with the current technology (Gemini screwed up big time upon release, just as Bard did before it).
Under the hood of any business, a large number of small processes work in tandem to achieve a well-defined outcome. Trying to use large generative AI to automate each process is not much different from hiring a coach to transport a single passenger – assuming the generative AI wont screw up the task.
In fact, the biggest value by far comes from “conventional” supervised AI, according to Andrew Ng. These models are narrow in scope and small in size, making them manageable and efficient.
That’s why, for our property valuation platform, AccuVal, we train deep learning regression models to predict the value of any given house in Britain (even if the house doesn’t actually exist!). The accuracy it achieves is miles ahead of the closest competitor (they use old fashioned AVM). It also costs a fraction to train, but more importantly, costs peanuts to run in production (and it takes mere milliseconds to make each inference on CPUs).
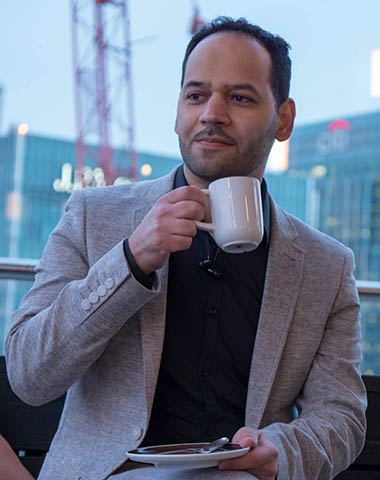
Jaafar Almusaad is a co-founder and CTO of REXSMART. Jaafar has over two decades’ experience in computer engineering, software development and data science. He holds a Master’s degree in Information Technology Management from the University of Sunderland.
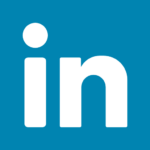